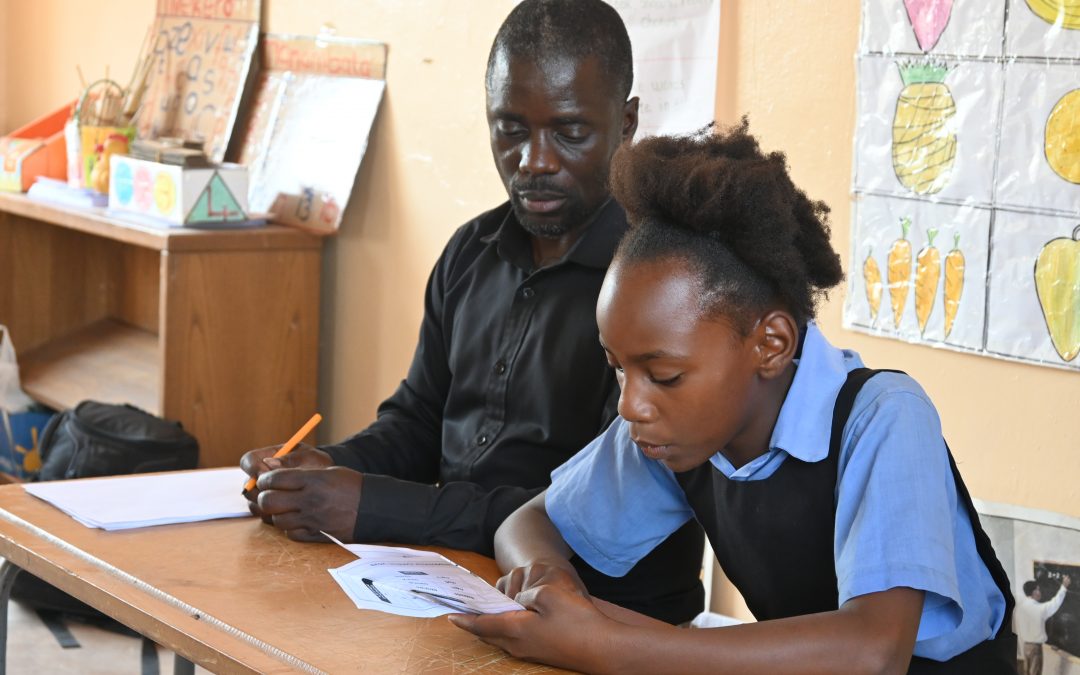
A teacher assesses a learner during a TaRL session in Zambia. (Photo: TaRL Africa)
As collecting and reviewing data at a large scale becomes increasingly possible, Foundational Literacy & Numeracy (FLN) programmes worldwide are turning to data to learn about programme achievements and gaps. Data — with its ability to tell a story — widens our worldview and empowers us to make informed decisions to improve programmes.
For those of us working in Measurement, Learning & Evaluation (MLE), high-quality data is the foundation we strive for. This is because our stories are only as strong as our data — if there are issues with the data quality, it may mean that the findings that the data generates are inaccurate. With their toolkit of spot checks, back checks, high-frequency checks, and independent data collection, MLE units attempt to build an edifice of robust, reliable and valid data.
However, when data is entwined with the programme, then strengthening the data also means strengthening programme implementation; the two are not separate issues. By aligning measurement with the programme and building systems that implementers can use easily, we can embark on a sustainable path to better quality data.
Learning about FLN Skills with Assessments Data at TaRL Africa
In Teaching at the Right Level (TaRL) programmes, the people collecting the (assessment) data are school teachers, head teachers, government mentors, and statisticians. They gather assessment data for all the learners three times over the course of a programme — at the start (baseline), middle (midline) and end (endline).
From formative assessments to data
Left: A teacher assessing a child one-on-one in Kebbi, Nigeria (Photo: Afees Odewale, TaRL Africa).
Right: Assessment results of all the school children on display in the Head Teacher’s Office in Southern Province, Zambia (Photo: Apoorva Baheti, TaRL Africa)
In TaRL programmes, data is used in diverse ways. In the classroom, teachers use assessment data to gauge the learning levels of their learners and tailor instruction to their respective learning needs. For mentors, the data tells them which schools are progressing well and which ones may need more visits and support. The data also makes the programme’s progress and achievements visible to stakeholders internally and externally, moving them to focus on certain geographies, subjects, and learning levels.
Alas, all these wonderful uses of learning data are possible only if the data is accurate.
Ensuring High-Quality Data: A Programme-Centric Approach
Data collected as a part of implementation is different from data collected for pure research. Even though both implementers and researchers are keenly interested in knowing if their data quality is good, the subsequent steps for the two are different.
- While data is the star of the show in research (and large budgets dedicated to it are justifiable), it is only a powerful supporting piece in an FLN programme. Every dollar spent on better data quality is a dollar taken away from materials, teacher training, and mentor support. Any means to measure and improve data quality, therefore, must not be too expensive.
- Data from the programme streams in continuously, term after term and year after year. It is not a one-off or short-term affair. A one-time fix to data quality issues is not enough, so solutions must be sustainable.
- Finally, programme data is gathered by the same people who implement the programme (i.e., teachers and mentors). Therefore, solving the issues (particularly at scale) will require understanding the challenges and getting the buy-in and support of these key programme people.
If data collected for research is of low quality, the data collection process has to be fixed, perhaps by re-collecting the data, possibly through different enumerators, or a modified interview process.
Meanwhile, if data from a programme like TaRL is low quality, it is the programme that has to be strengthened because the data is embedded within it, and a good programme is a necessary precondition for good data. In such a scenario, the question to answer should not be, “How can we collect better quality data this time?” but rather “How can we strengthen systems in cost-effective ways to generate good quality data now and in future?”.
Systems strengthening first requires a complete understanding of where the gaps and weaknesses lie within the system. In our case, it requires going into schools and speaking with teachers, mentors and relevant government officials at different levels to understand how people are interacting with the data processes and what challenges they face.
Specifically: Why would a teacher not submit accurate assessment data? Possible reasons could fall into any of the following categories:
By understanding which of these reason(s) is/are true in a given context, we reach one step closer to getting high-quality FLN data that can be used for action.
Arriving at Solutions
Sometimes, the remedy for data quality issues is purely a data systems fix, such as simplifying data recording forms, building in validation, introducing new training modules, sensitizing teachers, or increasing monitoring during assessments.
However, at the end of the day, it is possible that all of these steps are taken but data quality stays unsatisfactory. The simplest, smoothest functioning and most useful assessments will not be done well, for example, by demotivated teachers who do not understand the importance of assessment data.
In this way, what started as a data problem might actually be an implementation challenge and the issue may then no longer fall within the MLE jurisdiction alone. It may need a programmatic solution far beyond the scope of data checks. It may need collective thinking from all the different functions of the implementing organization or government on broader issues: How can the programme be designed to improve and sustain teacher motivation? Should teachers be given non-monetary incentives? Should the community be involved to hold teachers accountable?
Data is only the beginning of the conversation and data quality is only a symptom. Pulling the thread of data quality can set off a process of diving deeply into complex programmatic realities. From there, we begin to untangle what is working well and what needs attention and response in order to keep the programme on track to scale successfully. Asking the ‘why’ questions about data quality allows us to identify programme challenges, delve deep into them, and come together to try things that we haven’t before in our quest to truly make an impact.
What should I do if I would like to verify the data for my zone?